In the rapidly evolving digital world, the term ‘data analyst’ is not just a job title, it’s a way of thinking. While buzzwords like machine learning, big data, and AI grab headlines, the heart of data analysis lies in making astute, well-informed decisions. Imagine a data analyst as a chef in a kitchen of information: the ingredients are the data, and the meals are the insights gleaned. But how does one cook up a storm of intelligent decisions? It boils down to understanding the four fundamental levels of analytics: descriptive, diagnostic, predictive, and prescriptive.
Descriptive Analytics: The ‘What Happened?’
Descriptive analytics, the starting block in the data analytics relay, is where most organizations spend a chunk of their time. Think of it as the ‘historian’ of data analysis. It’s all about looking back and understanding what happened. For instance, a retail company might use descriptive analytics to understand last quarter’s sales trends. But it’s not just about basking in the glory of past data. A proficient data analyst knows that while descriptive analytics sets the scene, it’s just the appetizer in the full course meal of analytics. Moving beyond mere observation to extract meaningful insights is where the journey truly begins.
Descriptive analytics plays a crucial role in the world of data analysis by providing a clear picture of past performances and events. Here are some examples to illustrate its application in various sectors:
- Retail Sales Analysis: A retail store uses descriptive analytics to evaluate sales data from the past year. This analysis can reveal trends in which products were bestsellers, what times of year sales peaked, and which locations had the highest sales volume. This information is vital for inventory management, marketing strategies, and understanding consumer behavior.
- Website Traffic Reports: For online businesses, descriptive analytics is used to assess website traffic. Tools like Google Analytics can provide reports showing the number of visitors, page views, bounce rate, average time spent on the site, and the sources of traffic. This data helps in understanding user engagement and effectiveness of online content.
- Healthcare Patient Data: Hospitals use descriptive analytics to track patient admissions over time. They can analyze data such as the number of patients treated, the most common ailments, average length of stay, and patient outcomes. This information is crucial for resource allocation, understanding public health trends, and improving patient care services.
- Financial Performance Reports: Companies use descriptive analytics for financial reporting. This involves analyzing past financial data like revenues, expenditures, profit margins, and cash flow. These reports are essential for stakeholders to understand the financial health and performance of the business over a specific period.
- Social Media Analytics: Businesses analyze social media engagement through descriptive analytics. Metrics such as likes, shares, comments, and follower growth on platforms like Facebook, Twitter, or Instagram offer insights into brand reach, audience engagement, and content performance.
- Customer Satisfaction Surveys: Companies often use descriptive analytics to summarize responses from customer satisfaction surveys. By analyzing data on customer feedback, businesses can gauge overall satisfaction levels, identify areas of improvement, and understand customer needs and preferences.
- Supply Chain Management: Descriptive analytics helps in monitoring supply chain and logistics operations. Companies can track metrics like shipping times, inventory levels, and supplier performance to ensure efficient operation and identify potential areas of improvement.
Each of these examples showcases how descriptive analytics serves as a foundational tool, helping businesses and organizations understand their current situation and historical performance. This understanding is essential for informed decision-making and strategic planning, laying the groundwork for more advanced analytical techniques like diagnostic, predictive, and prescriptive analytics.
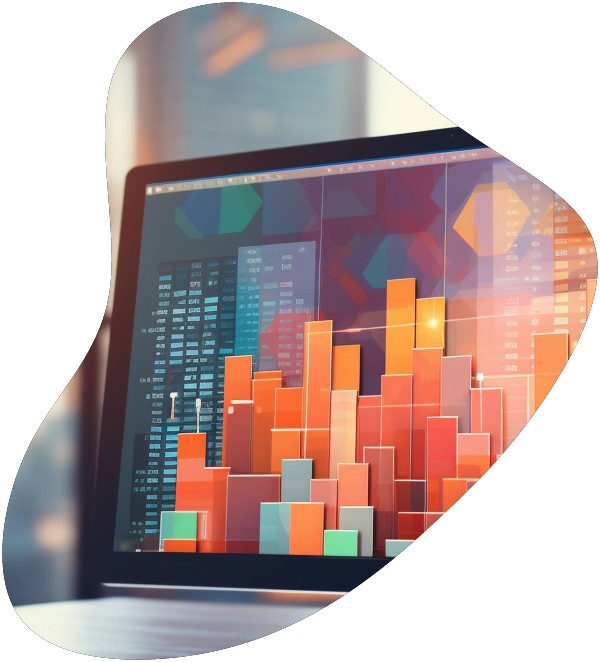
Data Analyst Career Path
Elevate your career with our Data Analyst Training Series. Master SQL, Excel, Power BI, and big data analytics to become a proficient Data Analyst. Ideal for aspiring analysts and professionals seeking to deepen their data skills in a practical, real-world context.
Diagnostic Analytics: The ‘Why Did It Happen?’
Now, enter diagnostic analytics, the ‘detective’ phase. This is where data analysts don their detective hats and dive into the data to unearth the reasons behind the observed trends. Let’s say our retail company noticed a dip in sales. Diagnostic analytics steps in to analyze why. Was it due to a new competitor? A change in customer preferences? Or perhaps a failed marketing campaign? It’s like visiting a doctor who doesn’t just note the fever but investigates the cause, prescribing a remedy. In the world of data, this phase is crucial for understanding the underlying stories behind the numbers.
Diagnostic analytics takes a deeper dive into data to understand the reasons behind certain trends or events identified during the descriptive analysis phase. Here are some real-world examples of how diagnostic analytics is applied across various sectors:
- E-commerce Shopping Cart Abandonment Analysis by Data Analysts: When an online retailer notices a high rate of shopping cart abandonment, data analysts play a crucial role. They delve into customer behavior data to diagnose why customers are leaving without completing their purchases, uncovering factors like unexpected shipping costs or complex checkout processes.
- Manufacturing Process Optimization with Data Analysts: In a manufacturing company, data analysts are key in diagnosing the causes of production delays or quality issues. They analyze data from various stages of the manufacturing process to identify issues such as equipment malfunction or supply chain disruptions, providing insights for process improvements.
- Healthcare Readmission Rates and Data Analysts’ Role: To tackle higher than average patient readmission rates in a hospital, data analysts examine patient records and treatment protocols. Their analysis helps in identifying patterns among readmitted patients, such as premature discharge or specific treatment complications, leading to better patient care strategies.
- Telecom Customer Churn Analysis by Data Analysts: In the telecom sector, data analysts are instrumental in understanding the spike in customer churn. They analyze call center data, service usage patterns, and customer feedback to diagnose factors contributing to customer dissatisfaction, aiding in customer retention strategies.
- Urban Traffic Congestion Studies by Data Analysts: City planners often rely on data analysts to diagnose causes of traffic congestion. By analyzing traffic flow data and accident reports, data analysts help identify specific bottlenecks, providing insights for effective traffic management planning.
- Energy Consumption Diagnostic by Data Analysts in Buildings: Facility managers work with data analysts to diagnose reasons for high energy usage in buildings. Data analysts examine systems and occupancy patterns to pinpoint inefficiencies like poor insulation or unnecessary energy consumption, leading to energy-saving measures.
- Marketing Campaign Effectiveness and Data Analysts: For a marketing campaign with lower than expected engagement, data analysts dissect various elements like email open rates and social media interactions. Their diagnostic analytics identify successful aspects and areas needing improvement, guiding future marketing strategies.
In each instance, the role of a data analyst is pivotal in conducting diagnostic analytics. They not only help in identifying the problems but also provide data-driven insights that are crucial for informed decision-making and strategic planning in various industries.
Predictive Analytics: The ‘What Could Happen?’
Next up is predictive analytics, the ‘fortune teller’ of data. This level is akin to gazing into a crystal ball but with data-driven precision. Predictive analytics uses historical data, statistical algorithms, and machine learning techniques to forecast future outcomes. Imagine our retail company using predictive analytics to foresee seasonal sales trends or customer buying habits. This foresight enables the company to stock up adequately, plan marketing strategies, or even adjust pricing in anticipation. However, predictive analytics is like a high-level chess game; it requires not just data but the skill to interpret it effectively. It’s a critical tool in the data analyst’s arsenal, allowing organizations to prepare for the future rather than just react to the past.
- Retail Sales Forecasting by Data Analysts: Data analysts in a retail company use predictive analytics to forecast future sales trends. By analyzing historical sales data, customer demographics, and market trends, they can predict which products are likely to be in high demand in the upcoming season, aiding in inventory management and marketing strategies.
- Financial Risk Assessment with Data Analysts: In the financial sector, data analysts employ predictive analytics to assess credit risk. By examining past transaction histories, repayment patterns, and economic factors, they can predict the likelihood of defaults or delinquencies, which helps in making informed lending decisions.
- Healthcare Predictions by Data Analysts: In healthcare, data analysts use predictive analytics to anticipate patient outcomes. By analyzing patient data, treatment histories, and clinical parameters, they can predict the likelihood of readmissions or identify patients at risk of developing certain conditions, enabling proactive care management.
- Predictive Maintenance in Manufacturing by Data Analysts: Data analysts in manufacturing industries utilize predictive analytics for equipment maintenance. By analyzing machine performance data and historical maintenance records, they can predict when a machine is likely to fail or require maintenance, thus preventing downtime and saving costs.
- Customer Churn Prediction by Data Analysts: Data analysts in telecommunication companies use predictive analytics to identify customers who are likely to churn. By analyzing usage patterns, customer service interactions, and billing history, they can predict which customers are at risk and develop targeted retention strategies.
- Traffic Flow Predictions by Data Analysts in Urban Planning: Data analysts in urban planning use predictive analytics to forecast traffic patterns. By analyzing historical traffic data, urban growth trends, and event schedules, they can predict potential traffic hotspots and congestion, aiding in traffic management and infrastructure planning.
- Predictive Policing with Data Analysts in Law Enforcement: In law enforcement, data analysts use predictive analytics to forecast crime trends. By analyzing past crime data, demographic information, and social factors, they can identify areas with a higher likelihood of crime, helping law enforcement agencies allocate resources more effectively.
In each of these examples, the data analyst plays a crucial role in harnessing the power of predictive analytics. By interpreting historical data and identifying patterns, they provide valuable foresights that help organizations in various sectors to anticipate future trends, mitigate risks, and make strategic decisions.
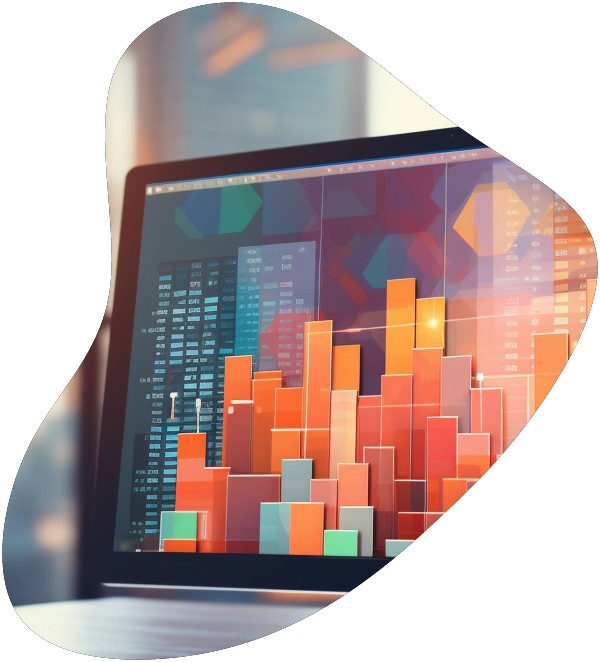
Lock In Our Lowest Price Ever For Only $16.99 Monthly Access
Your career in information technology last for years. Technology changes rapidly. An ITU Online IT Training subscription offers you flexible and affordable IT training. With our IT training at your fingertips, your career opportunities are never ending as you grow your skills.
Plus, start today and get 30 days for only $1.00 with no obligation. Cancel anytime.
Prescriptive Analytics: The ‘What Should We Do?’
Finally, we arrive at prescriptive analytics, the ‘strategist’ of the bunch. This level takes the insights gained from all previous stages and prescribes specific actions. It’s like having a GPS for business strategy. Using our retail example, prescriptive analytics might suggest the best time to launch a new product line or the ideal marketing channel to engage customers. It combines the power of descriptive, diagnostic, and predictive analytics to not just predict the future but to shape it. This phase is where data analysis transcends from being informative to being transformative, guiding businesses towards optimal decision-making.
Prescriptive analytics is a powerful branch of advanced analytics that focuses on providing specific recommendations and actions to optimize outcomes. Data analysts play a crucial role in prescriptive analytics by leveraging their skills to extract, analyze, and interpret data. Here are some examples of how prescriptive analytics,
- Enhancing Data Analyst Productivity: Prescriptive analytics tools can assist data analysts by automating repetitive tasks such as data cleansing and preprocessing. By doing so, data analysts can focus on more strategic aspects of their work, such as developing predictive models or generating actionable insights.
- Optimizing Resource Allocation for Data Analysts: Prescriptive analytics can help organizations allocate their data analyst resources more effectively. By analyzing historical workloads and project priorities, it can recommend the allocation of data analysts to projects that have the highest potential impact.
- Data Analyst Skill Development: Prescriptive analytics can identify skill gaps among data analysts based on their performance and project outcomes. Recommendations for training or skill development can be provided to ensure that data analysts have the necessary expertise to excel in their roles.
- Customized Data Analysis Workflows: Prescriptive analytics platforms can create customized data analysis workflows for data analysts. These workflows can include recommendations on which data sources to use, which algorithms to apply, and how to visualize and present the results effectively.
- Data Analyst Collaboration: Prescriptive analytics can facilitate collaboration among data analysts by recommending team compositions based on individual strengths and expertise. It can also suggest communication strategies and tools for effective teamwork.
- Predictive Maintenance for Data Analyst Tools: In the context of data analysis tools and software, prescriptive analytics can be used to predict when these tools may require maintenance or updates. This ensures that data analysts have access to reliable and up-to-date software.
- Optimizing Data Analyst Workloads: Prescriptive analytics can recommend workload balancing for data analysts, ensuring that they are not overloaded with tasks or underutilized. This helps in maximizing their productivity and job satisfaction.
- Improving Data Analyst Hiring Decisions: When it comes to expanding a data analytics team, prescriptive analytics can assist in making informed hiring decisions. It can analyze the existing team’s composition and skills to recommend the most suitable candidates for new positions.
- Cost Optimization for Data Analyst Activities: By analyzing the costs associated with data analysis projects, prescriptive analytics can suggest cost-saving measures, such as outsourcing certain tasks or leveraging open-source tools, to ensure efficient budget utilization.
- Data Analyst Performance Evaluation: Prescriptive analytics can provide a data-driven approach to evaluating the performance of data analysts. It can recommend key performance indicators (KPIs) and metrics that reflect their contributions to the organization’s objectives.
In summary, prescriptive analytics empowers data analysts by providing actionable recommendations and insights that can enhance their productivity, skills, collaboration, and overall contribution to an organization’s data-driven decision-making processes.
Harmonizing the Four Levels for Holistic Insights
In the realm of data analysis, these four levels are interconnected pieces of a larger puzzle. Each level has its unique value, but when combined, they provide a comprehensive view of the business landscape. The journey from descriptive to prescriptive analytics is like assembling a jigsaw puzzle. You start by laying out all the pieces (descriptive), figure out how they connect (diagnostic), envision what the completed puzzle looks like (predictive), and finally, put the pieces together to complete the picture (prescriptive). A data analyst who can navigate through all four levels is akin to a maestro conducting an orchestra, ensuring every instrument (data) plays its part in harmony.
The Role of Data Literacy and Team Skills
To effectively utilize these four levels of analytics, an organization must cultivate data literacy across its teams. It’s not just about having a few data analysts; it’s about embedding a data-driven mindset throughout the organization. Employees at all levels should be comfortable interpreting data and making informed decisions. This requires ongoing training and a culture that values data as a key asset. It’s about transforming data from a siloed specialty into a universal language spoken across the company.
Tying Analytics to Business Objectives and Goals
The ultimate aim of mastering these four levels of analytics is to align them with the organization’s key business objectives and goals. Data analysis is not just an academic exercise; it’s a strategic tool for driving business growth and innovation. By effectively leveraging descriptive, diagnostic, predictive, and prescriptive analytics, organizations can make well-informed decisions that propel them towards their long-term vision and objectives.
Tying analytics to business objectives and goals is crucial for organizations to ensure that data-driven insights and actions align with their strategic priorities. Here are some examples of how analytics can be connected to specific business objectives and goals:
- Increasing Revenue: A retail company can use sales data analytics to identify which products are selling well and strategically promote them to boost revenue. Analytics can help identify cross-selling and upselling opportunities to increase the average transaction value.
- Reducing Customer Churn: A subscription-based service provider can analyze customer behavior data to identify patterns leading to churn. By proactively addressing these issues and offering personalized incentives or solutions, they can reduce customer churn and increase customer retention rates.
- Improving Customer Satisfaction: Analytics can be used to analyze customer feedback, sentiment analysis, and customer support data to identify areas where customer satisfaction can be improved. Actionable insights can guide improvements in customer service, product features, or user experience.
- Cost Reduction: An organization can utilize cost analytics to identify areas of inefficiency or excessive spending. This may involve optimizing supply chain operations, renegotiating vendor contracts, or implementing energy-saving measures to reduce operational costs.
- Market Expansion: Analytics can help identify new market opportunities by analyzing demographic, geographic, and market trend data. Businesses can use this information to target expansion efforts in regions with high growth potential.
- Product Development and Innovation: By analyzing market research data, customer feedback, and competitive intelligence, businesses can align their product development efforts with market demand. This ensures that new products and features meet customer needs and preferences.
- Enhancing Employee Productivity: Workforce analytics can identify areas where employee productivity can be improved. This might involve optimizing work schedules, providing additional training, or implementing collaboration tools to boost team efficiency.
- Risk Management: Financial institutions can use analytics to assess credit risk and fraud detection. By identifying high-risk transactions or customers, they can take proactive measures to mitigate potential losses.
- Sustainability Goals: Organizations focused on sustainability can use environmental and energy consumption data to track progress toward reducing their carbon footprint. Analytics can provide insights into areas where sustainability efforts can be improved.
- Supply Chain Optimization: Analytics can optimize supply chain operations by analyzing data related to inventory management, demand forecasting, and supplier performance. This ensures efficient inventory levels and timely deliveries, reducing costs and improving customer satisfaction.
- Marketing ROI: Marketing analytics can tie marketing efforts to specific business objectives, such as customer acquisition or lead generation. By tracking the ROI of marketing campaigns, organizations can allocate resources to the most effective channels and strategies.
- Compliance and Regulatory Goals: Analytics can assist organizations in ensuring compliance with industry regulations and standards. By monitoring data for compliance violations and conducting regular audits, businesses can avoid costly penalties.
- Quality Improvement: Manufacturing companies can use analytics to monitor product quality and identify defects or issues early in the production process. This leads to improved product quality and reduced waste.
- Customer Lifetime Value (CLV): Businesses can calculate CLV using analytics to understand the long-term value of customers. This information can guide decisions on customer acquisition and retention strategies.
- Profit Margin Optimization: Analytics can analyze cost structures and pricing strategies to optimize profit margins. By identifying opportunities for cost reduction and pricing adjustments, businesses can improve profitability.
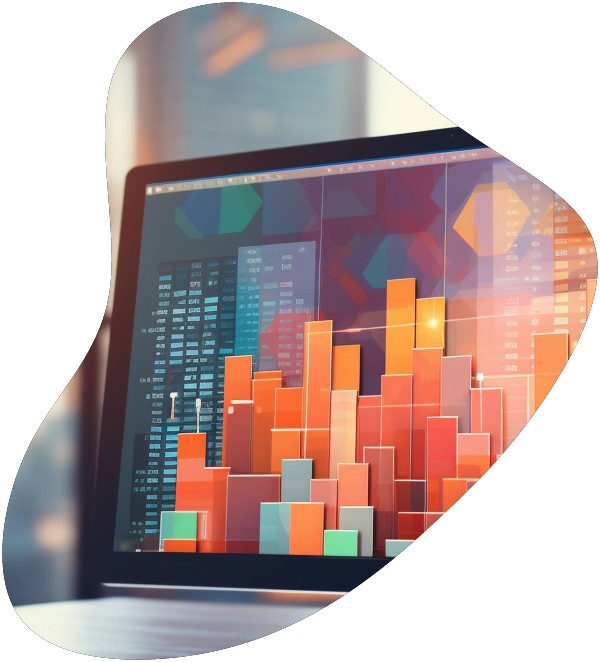
Data Analyst Career Path
Elevate your career with our Data Analyst Training Series. Master SQL, Excel, Power BI, and big data analytics to become a proficient Data Analyst. Ideal for aspiring analysts and professionals seeking to deepen their data skills in a practical, real-world context.
In each of these examples, analytics plays a vital role in helping organizations align their data-driven insights and actions with specific business objectives and goals, ultimately contributing to business success and growth.
Conclusion
In conclusion, the four levels of analytics – descriptive, diagnostic, predictive, and prescriptive – are not just steps but a journey towards mature, data-driven decision-making. Like a well-oiled machine, each part plays a vital role in understanding and leveraging data effectively. A data analyst is the engineer of this machine, ensuring each component works seamlessly to provide actionable insights. Remember, in the fast-paced digital world, data is the new currency, and analytics is the key to unlocking its true value. By mastering these four levels, organizations can turn data into a strategic asset, driving innovation and success in an increasingly data-centric world. In essence, data analytics is not just about processing numbers; it’s about weaving stories from data that guide business strategies and illuminate the path to growth and efficiency.
Frequently Asked Questions About Data Analysis Levels
What are the four levels of data analysis?
The four levels of data analysis are Descriptive Analysis, Diagnostic Analysis, Predictive Analysis, and Prescriptive Analysis. These levels represent a progression from basic data reporting to advanced decision-making and optimization.
What is the purpose of Descriptive Analysis?
Descriptive Analysis focuses on summarizing and presenting historical data to provide insights into past trends and patterns. Its primary purpose is to answer the question “What happened?” and provide a baseline understanding of data.
How does Diagnostic Analysis differ from Descriptive Analysis?
While Descriptive Analysis explains historical data, Diagnostic Analysis goes a step further to determine why certain events or trends occurred. It aims to identify the root causes behind specific outcomes and patterns.
What is the main goal of Predictive Analysis?
Predictive Analysis is all about forecasting future events or trends based on historical data and statistical modeling. Its primary goal is to answer questions like “What is likely to happen in the future?” by making data-driven predictions.
How does Prescriptive Analysis add value to decision-making?
Prescriptive Analysis not only predicts future outcomes but also provides actionable recommendations on what actions to take to achieve desired outcomes. It helps decision-makers by suggesting the best course of action to optimize results based on data and objectives.